Main Page
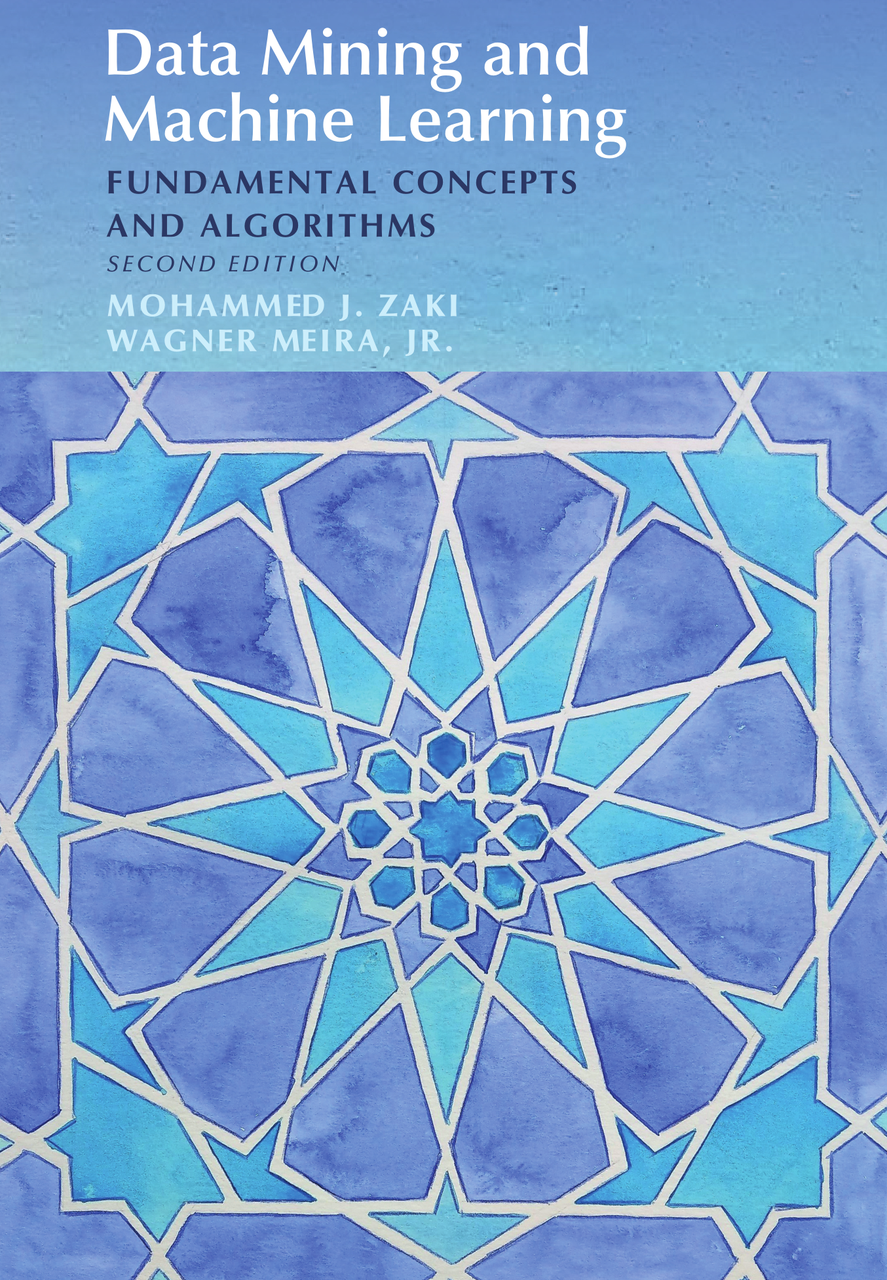
Description
The fundamental algorithms in data mining and machine learning form the basis of data science, utilizing automated methods to analyze patterns and models for all kinds of data in applications ranging from scientific discovery to business analytics. This textbook for senior undergraduate and graduate courses provides a comprehensive, in-depth overview of data mining, machine learning and statistics, offering solid guidance for students, researchers, and practitioners. The book lays the foundations of data analysis, pattern mining, clustering, classification and regression, with a focus on the algorithms and the underlying algebraic, geometric, and probabilistic concepts. New to this second edition is an entire part devoted to regression methods, including neural networks and deep learning.
This second edition has the following new features and content:
New part five on regression: contains chapters on linear regression, logistic regression, neural networks (multilayer perceptrons), deep learning (recurrent and convolutional neural networks), and regression assessment.
Expanded material on ensemble models in chapter 24.
Math notation has been clarified, and important equations are now boxed for emphasis throughout the text.
Geometric view emphasized throughout the text, including for regression.
Errors from the first edition have been corrected.
You can find here the online book, errata, table of contents and resources like slides, videos and other materials for the new edition.
Description of the first edition is also available.